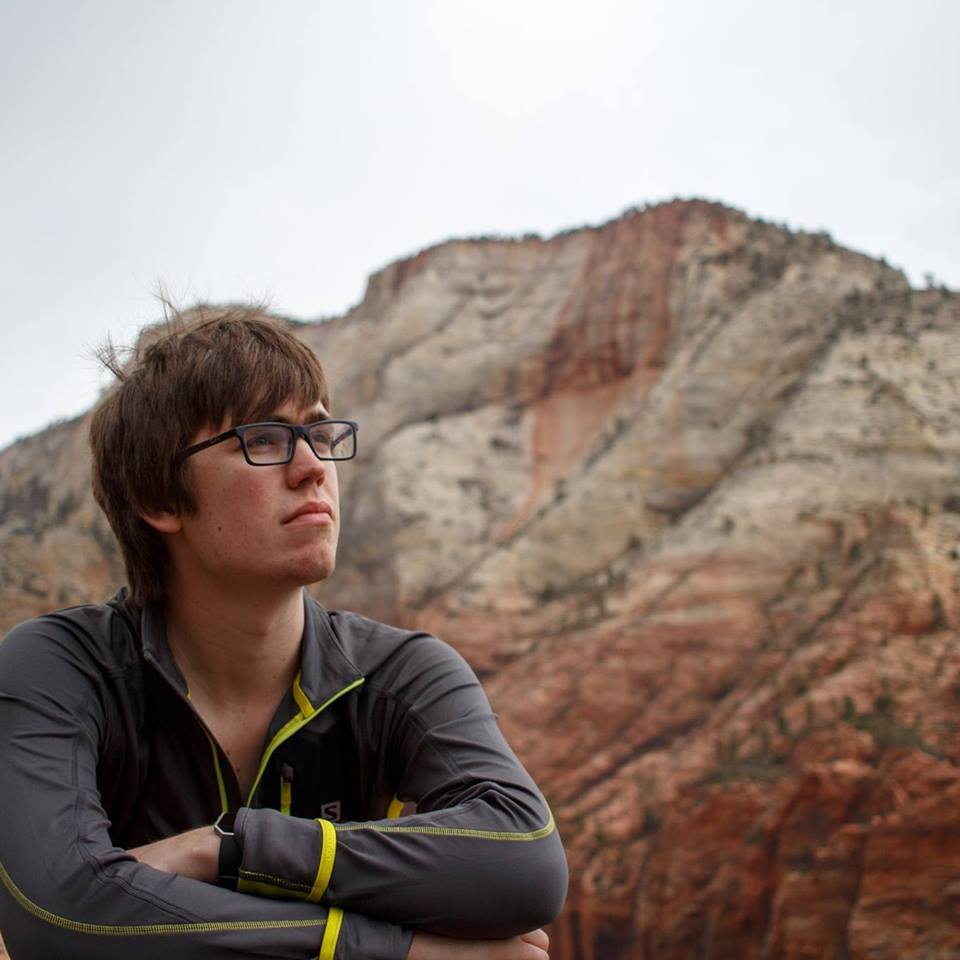
Davis Foote
Davis Foote is a student in EECS and Math at UC Berkeley. He has been a TA/head TA for CS 188 three times in addition to previously being on staff for CS 61A: Intro to CS, CS 70: Discrete Math and Probability, and CS 170: Algorithms and Complexity and teaching some workshops (mainly on functional programming) for the student-run organization Hackers@Berkeley. His current research is under Professor Pieter Abbeel and focuses on neural optimization techniques for reinforcement learning. Previous areas have included algebraic path problems and reinforcement learning problems with conflicting objectives. Prior to all of that, he spent most of his time playing guitar in a prog metal band before selling out and going to school.